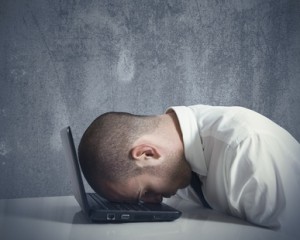
“No tears in the writer, no tears in the reader.
No surprise in the writer, no surprise in the reader.”
— Robert Frost
My first real programming language was Pascal, actually Turbo Pascal.
Turbo Pascal was not just Pascal — it was Pascal on steroids: small, fast, and yet affordable to everyone, including students and hobbyists. While the version numbers increased from 1.0 to 6.0 I wrote dozens of little tools, games and applications. For a long time, I had seen no need to switch to a crude, low-level language like C; Pascal had so many advanced features like a strong type system and sets.
One of the features I really loved was the ‘in’ operator, that allowed to test for set membership:
|
type days = (Sunday, Monday, Tuesday, Wednesday, Thursday, Friday, Saturday); |
|
var allday : set of days; workday : set of Monday .. Friday; today : days; |
|
begin ... if today in workday then writeln('I have to go to work today...') end ... |
Having an ‘in’ operator at your disposal means that there is no need to write lengthy if-then-else or switch-case abominations — you can express your intentions with ease and elegance.
I got so accustomed to Pascal’s sets and especially the ‘in’ operator that I missed it terribly in almost every programming language that I learned in the years to follow, including C, C++, Java, even Perl. I was really pleased to see that my next programming language — Python — had it.
But what was my point, again? Ah, yes…
The folks at E.S.R.Labs do an internal coding competition every once in a while. The current assignment has to do with finding best strategies for knocking-out your opponent in a Tron-like game. The game framework itself is based on Node.js/JavaScript but since I’m much more familiar with Python, I decided to do my algorithm prototyping in Python and later port everything back to JavaScript.
Actually, the transformation from Python to JavaScript was a lot easier than I thought, still my JavaScript version didn’t work as expected. After some (read: way too much) debugging, I found the problem; later, I decided that I had to write a blog post about it, as part of my therapy to get over my trauma.
Let’s first have a look at a snippet of the original Python code. I used the ‘in’ operator to check whether the value of ‘field’ at position ‘x’ and ‘y’ was either ‘FIELD_FREE’ or ‘FIELD_OTHER’:
|
# Go down. x = me_x + 1 y = me_y if y < len(field[x]) and field[x][y] in [FIELD_FREE, FIELD_OTHER]: to_visit.append(x) to_visit.append(y) if first_round: ... |
Now for the JavaScript version. As you will surely agree, it is almost identical:
|
// Go down. x = me_x + 1; y = me_y; if (x < field.length && field[x][y] in [Field.FREE, Field.OTHER]) { to_visit.push(x); to_visit.push(y); if (first_round) { ... |
But as we all know looks can be deceiving. With regards to the ‘in’ operator, the designers of the JavaScript language grossly violated the “Principle of Least Surprise“: Everyone (not just people with a Pascal background) would assume that the ‘in’ operator tests whether a particular value is contained in a given set. But not so in JavaScript — not at all! In JavaScript, the ‘in’ operator does what you would expect least.
In JavaScript the ‘in’ operator tests whether its left-hand argument is a valid index into the right-hand argument. That is, the expression
|
field[x][y] in [Field.FREE, Field.OTHER] |
would evaluate to true if the value at position (x,y) is an integer value in range [0..1], because only 0 and 1 are valid indices into a set (actually an array) containing just two elements. If the value at position (x,y) equaled the value of ‘Field.FREE’ or ‘Field.OTHER’ the result would be false, unless — by sheer coincidence and hard luck — the symbolic constants ‘Field.FREE’ and ‘Field.OTHER’ happen to have an integral value of either 0 or 1. Which they had in my case, at least in part:
|
Field = { FREE: 0, YOU: 1, OTHER: 2, WALL: 3 } |
Hence, the JavaScript version didn’t fail loudly and obviously, but in very subtle ways.
The main reason why it took me so long to track this bug down was that the last thing I questioned was the behavior of the ‘in’ operator. When I finally proved by debugging that ‘in’ worked different to my expectations, my initial thought was that there must have been a bug in my version of Node.js. But deep in my heart I knew that this was extremely unlikely. So I did a search on the Internet and found out about the shocking truth.
Why on earth did the designers of JavaScript implement the ‘in’ operator the way they did? It works kind of as expected with dictionaries (aka. associative arrays) but using a dictionary would have been an ugly and unnatural choice in my case. For regular arrays, the ‘in’ operator is worse than useless: it deludes programmers into using it but then doesn’t live up to their expectations.
Then, I would rather have no ‘in’ operator at all.